Synthetic Customer Data Enrichment
AI-powered customer feedback so you can take action now
Native AI turns insight into impact
Introducing Native AI, the always-on market intelligence platform that helps you understand, innovate, and create ideal experiences for your customers.
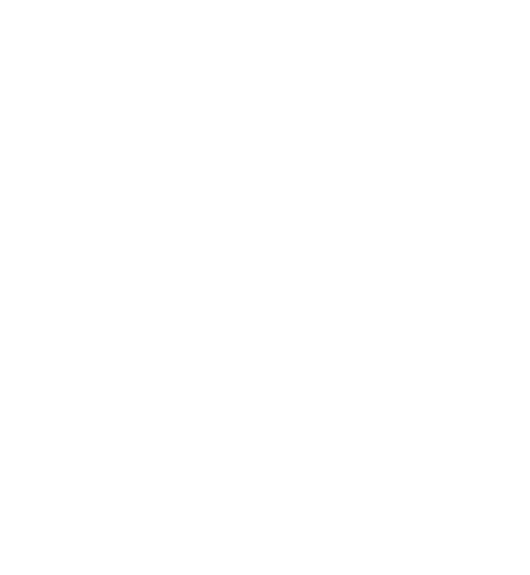
A better way to find the answers you need
Create impact through measurable improvements to products, customer experience, and marketing.
-
Analyze millions of qualitative and quantitative data points
-
Automate product and keyword tracking across the web
- Converse with digital clones of your customers in real-time
Insights Dashboard
Customize and export reports with full choice of visualizations. Set up alerts for specific products and keywords, and monitor trends over time in order to predict future consumer behaviors and identify white-space opportunities.
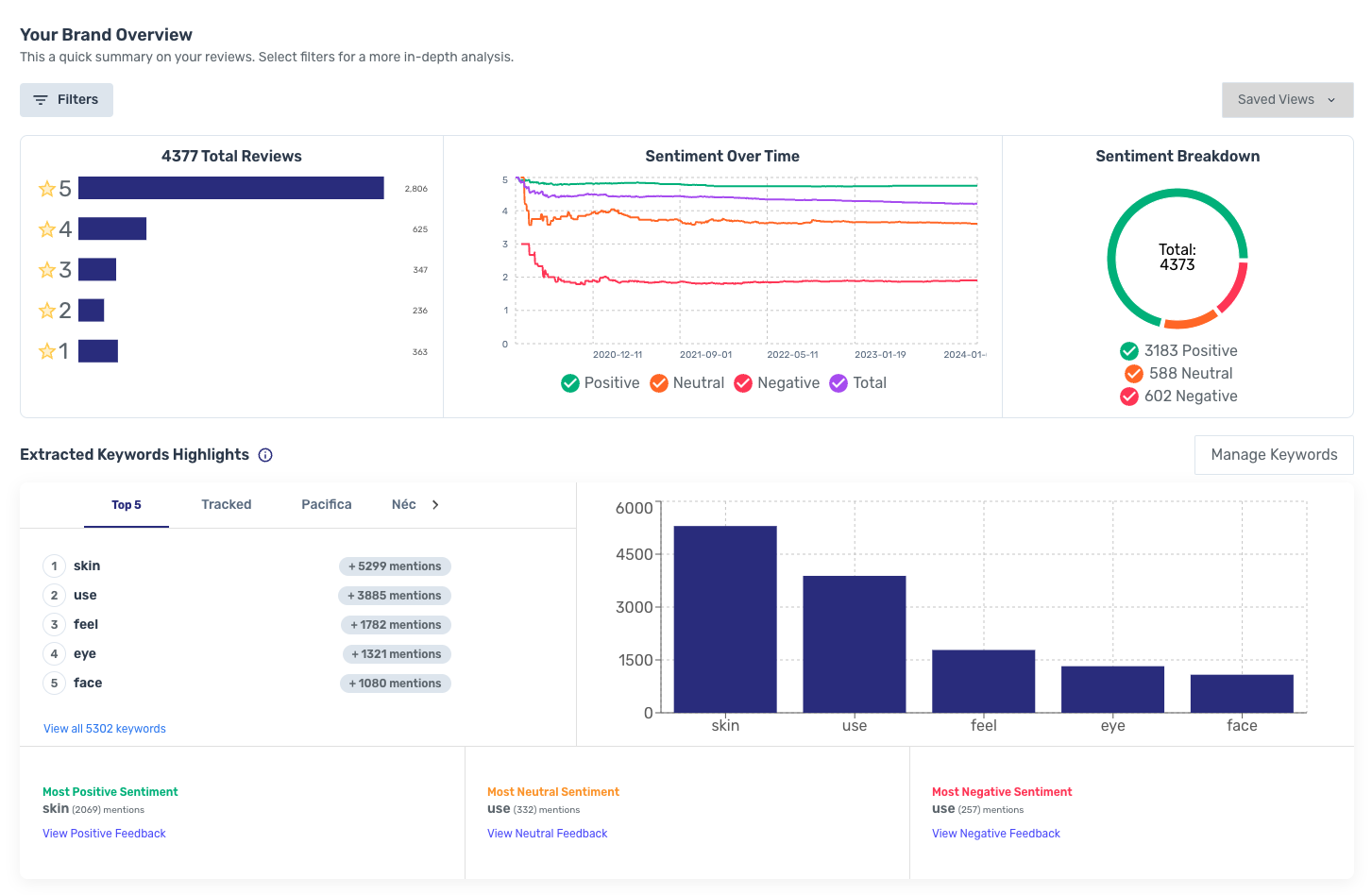
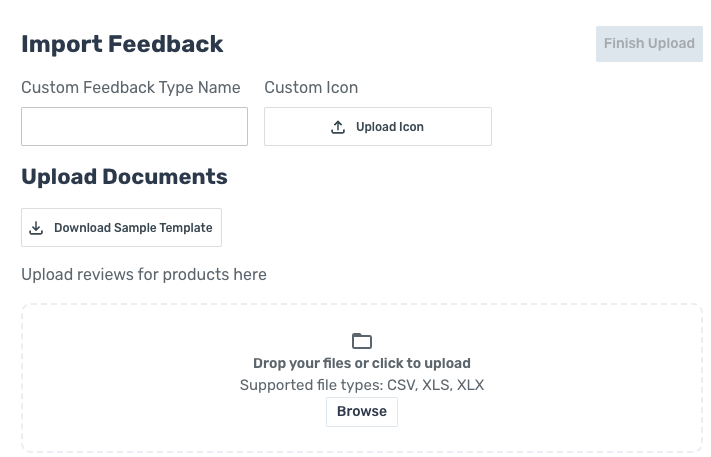
Bring Your Own Data
Upload your first party data in any structured or unstructured format. Our platform is compliant with international data processing regulations to eliminate unnecessary risk.
Digital Twins
Converse with digital clones of your customers or your competitors' customers. Filter by demographic, purchase channel, behavior, and more. Create visualizations and export your results.
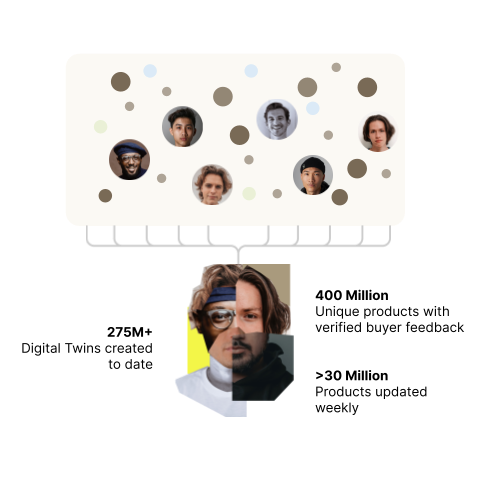
Expert White-Glove Service
Our platform is designed for you to be successful independently, but we're here to make sure you’re able to take full advantage of the value Native AI provides.
The Native AI Difference
We're not like other all-in-one insights platforms. Get a peek under the hood.
Data Safety & Privacy
Advanced Orchestration
Synthetic Output Controls
Synthetic Customer Data Enrichment
In the world of AI, synthetic customer data has emerged as a significant breakthrough. Leveraging advanced tools and methods, businesses can now generate synthetic data from real data, thereby furnishing a profound understanding of customer behaviors, preferences, patterns, and propensities.
By using such tools, organizations can construct enriched customer profiles that resemble real customer data, but do not contain any confidential customer information. These tools utilize advanced algorithms to create data that impeccably mimics the patterns, tendencies, and behaviors of actual customer data.
Implementing synthetic customer data enrichment can enhance the accuracy of customer behavior predictions and enable organizations to strategize their marketing initiatives more effectively. This is due to the data's ability to emulate real-life human behavior, making the data more relatable and realistic.
Best synthetic data generation tools, including Native AI, provide efficient ways of producing high-quality synthetic data that safeguard private information while giving businesses a realistic insight into their customer base. Investing in these tools can be a productive approach for organizations aiming to extract meaningful customer insights while adhering to data privacy norms and regulations.
In essence, synthetic data paves the way for data-driven opportunities without compromising data security. This modern age calls for a sufficient comprehension of synthetic data meaning and its potential application in various business sectors. The enrichment of synthetic customer data is not only a hallmark of technological advancement, but it underscores the significance of data privacy in today's digital world.
Synthetic Data Examples
Generating synthetic data is the process of creating artificial data algorithmically, rather than by collecting real-world data. This method is used when real-world data is either completely inaccessible or is difficult to procure due to privacy or ethical limitations. It plays a substantial role in diverse fields, especially in sectors where data privacy is a premium, such as healthcare, banking, and advertising.
Venturing deeper into the realm of artificial intelligence, synthetic data plays a huge role. Generative AI systems, for instance, can conjure realistic data resembling real-world scenarios, thus aiding in the training of complex AI and Machine Learning models. These include simulated images for computer-vision algorithms, artificial patient records for machine learning in healthcare, or fake credit card transactions to help train fraud-detection systems.
Put under the microscope synthetic data generation using generative AI is an increasingly viable solution for overcoming data limitations. Different from simulated data, synthetic data isn't necessarily based on explicit models of the real world. While simulated data merely mirrors existing processes or systems, synthetic data employs Generative AI techniques, such as Generative Adversarial Networks (GANs), that learn to mimic the distribution of real-world data.
Exploring synthetic data use cases, it's interesting to note that this technology is used for testing and validation in agile software development practices, enhancing cybersecurity strategies by creating a cyber range filled with synthetic data, or even for AI model development where usage of real data can infringe on privacy rights.
For programmers and data scientists, the programming language Python offers various tools and libraries to generate synthetic data. A widely popular choice is the SMOTE technique (Synthetic Minority Over-sampling Technique), which raises a minority class's numbers in an imbalanced dataset by generating synthetic samples. This allows Python programmers an alternative means to handle imbalanced classes, improve model performance, and ultimately revolutionize synthetic data to new fringe borders.
Synthetic Data Generators
Synthetic data generation is utilized by a multitude of companies globally, who are reaping the rewards of using artificially crafted data to enhance their operations, research, and applications.
While discussing synthetic data generation methods, one cannot overlook the role of machine learning. In particular, synthetic customer data enrichment has surfaced as a prominent technique within this domain. The process involves the creation of a simulated data set that precisely mirrors the attributes and statistical properties of original customer data. This is instrumental in providing a comprehensive and detailed set of insights, useful for predictive modeling, testing, and optimization exercises.
Just as a musician molds melodies to create symphonic harmonies, engineers develop algorithms to generate synthetic data for machine learning. It is a meticulous process that entails data analytics, sorting, clustering, and intelligent manipulation of data points.
One such example would be synthetic data generation using Generative AI. This employs advanced artificial intelligence algorithms to fabricate high-quality synthetic data which yield better machine-learning outcomes. The model uses intricate calculations, mathematical functions, and problem-solving operations to generate data indistinguishable from real datasets. This can become particularly useful in the fields of market research and consumer insights.
A host of these operations can be executed programmatically. For instance, synthetic customer data enrichment in Python has gained momentum due to its practicality and efficiency. Python offers a plethora of libraries and tools that enable data scientists to generate synthetic data seamlessly. It facilitates a smoother development cycle, capturing covert patterns and correlations within the data with detailed precision.
In encapsulating the entire discourse on synthetic data generators, it becomes clear how integral this phenomenon has become in the modern technological landscape. From tech giants to burgeoning start-ups, synthetic data generation is creating efficiency, leading to better real-world outcomes.